Intelligent, auto-optimizing plants: In-Trace – Markerless Component Identification
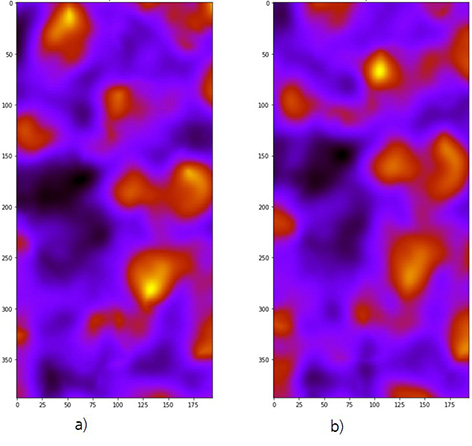
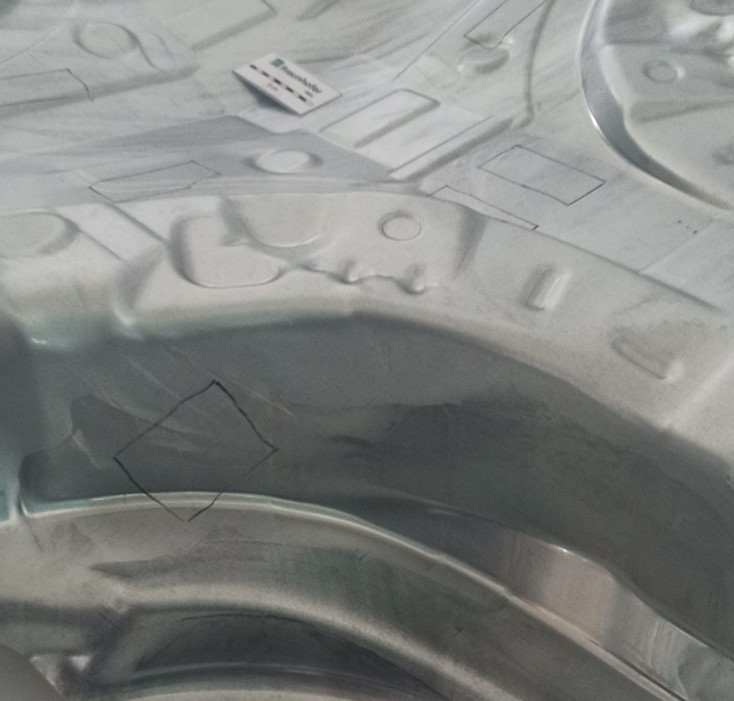
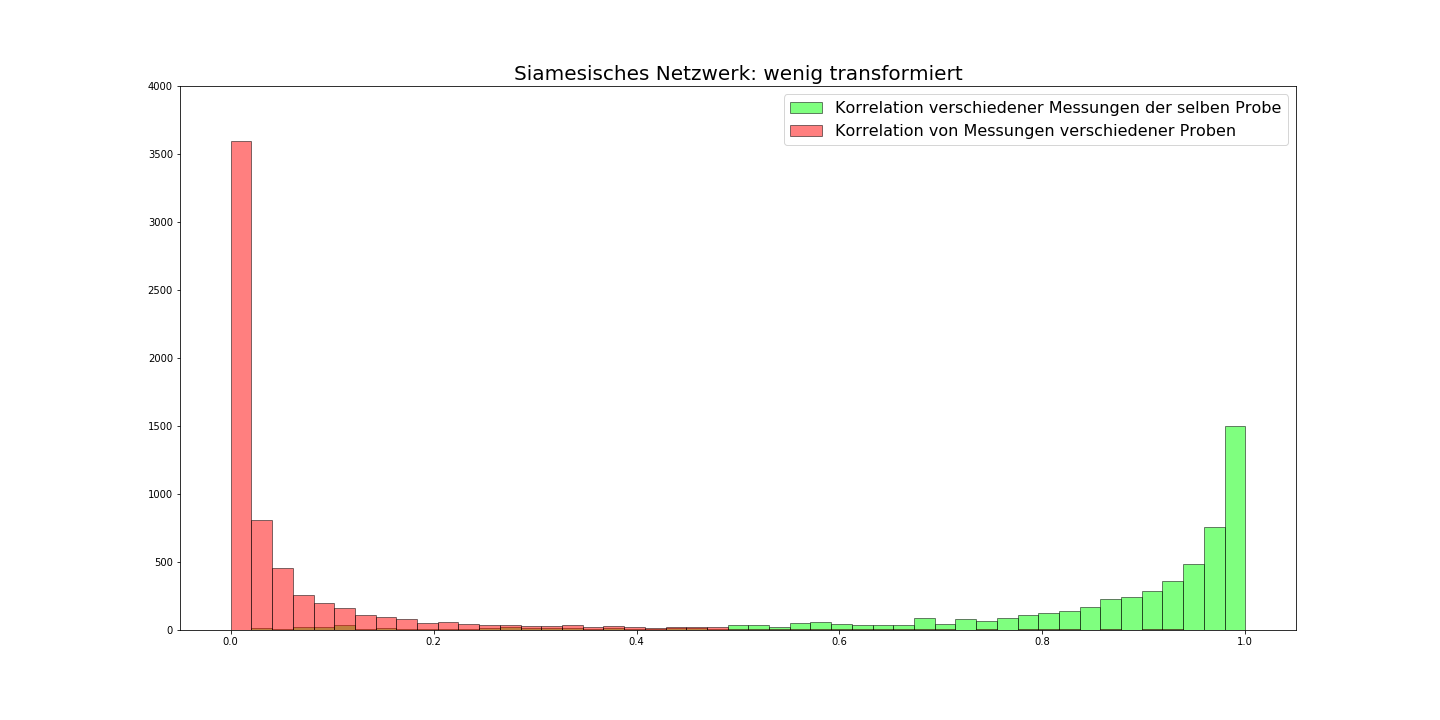
Reliable, cross-production identification of metallic components by means of their material fingerprint, based on intrinsic electromagnetic measurement variables
The distinct identification and complete traceability of each semi-finished product and component at any time during processing enables the optimization of product quality and resource efficiency. This is an essential prerequisite for the development of self-organizing value chains (I4.0). Due to the production process, conventional object markings, such as labels, barcodes, etc., usually cannot remain on the object, neither permanently nor undamaged. Optically detectably surface characteristics of a component can only be used until the surface is significantly changed by forming, machining or coating. In order to identify a component even after such process steps, new sensory processes are required which allow to detect features from the inside of the component. The point of focus of the “In-Trace“ project is a novel electromagnetic process which detects characteristic (micro-)structural intrinsic features of the material that remain the same even when the surface is changed.
Currently, a sensor system ready for industrial use is being developed. The system is based on an inductive sensor array, which is configured to measure a component area of approx. 50 x 60 mm2. The aim is to achieve a measurement time of no more than 0.5 seconds. The sensor-based identification data is restored in a feature space, representing the component as a unique individual. The resulting feature space is classified using suitable machine learning methods. Stable and partly redundant features enable identification even if only a part can be detected. The identification is supported by a digital object memory and the additional use of process and quality data in the feature space, which enable the prediction of feature changes. In this way, the influence of disturbance variables, such as changes in sensor lift-off, can be eliminated.
Fields of application / Possible uses
First, possible applications in sheet metal processing are examined. It has been demonstrated that the new traceability system can be used to identify a component even after it has been formed. This is also demonstrated for the acquisition of in-process measurement data in the production of rear light pots. To this purpose, the traceability system will be validated on an automated test rig in industrial conditions. Furthermore, the system will also be used for quality inspection of cast components.